Learning LLM Magic
The world of Generative AI has been pretty inescapable for a while, commercial models running on paid Cloud instances are everywhere. With your data stored securely on-prem in IRIS, it might seem daunting to start getting the benefit of experimentation with Large Language Models without having to navigate a minefield of Governance and rapidly evolving API documentation. If only there was a way to bring an LLM to IRIS, preferably in a very small code footprint....
Some warnings before we start
- This article targets any recent version of IRIS (2022+) which includes Embedded Python support. This should work without issue on IRIS Community Edition
- LLMs are typically optimised for use against GPU processing. This code will operate correctly against a CPU-only system, but it will be an order of magnitude slower compared to a system which can leverage a GPU
- This article uses fairly small Open Source models, to keep performance on less powerful hardward at a sensible level. If you have more resource, this tutorial will work on larger models without any major changes (just substitute the model name, in most cases)
Part 1 - Isn't hosting an LLM difficult?
The LLM ecosystem has evolved rapidly. Luckily for us, the tooling for this ecosystem has also evolved to keep pace. We are going to use the Ollama package. This can be installed on your platform of choice using their installation tools (available at https://ollama.com/) Ollama allows us to spin up an interactive session to begin using LLM models, but also allows for very easy to use programatic access via Python APIs. I am not going to cover installing Ollama in this article, but come back here when you have completed the install.
Excellent, you made it back! Time to spool up a model. We are going to use the reasonably lightweight Open Source GEMMA model, at the lowest entry point (2 billion) https://ollama.com/library/gemma:2b. With Ollama installed, running this is easy. We just need to run
ollama run gemma:2b
On our first run of this, the model will download (it's quite large, it might take a minute), install, and finally, you will get an interactive prompt into the LLM. Feel free to ask it a question to verify that it's operating correctly
.png)
We now have an LLM cached and available to use on our instance! Now, let's connect it to IRIS.
Step 2 - Accessing Ollama from IRIS to summarise text data
Before we begin, we will need to install the Ollama Python library. This will provide very easy and automated access to this Ollama instance and model. Refer to the documentation for your specific version to ensure you are running the correct installation command (https://docs.intersystems.com/irislatest/csp/docbook/DocBook.UI.Page.cls... is the current version). On my instance, I ran
python3 -m pip install --target /db/iris/mgr/python ollama
We are now ready to create a Business Operation which will use this library to access the model. Create a class which extends Ens.BusinessOperation and a Message classes to hold our requests and responses
Class Messages.SummaryInput Extends Ens.Request
{
Property jsonText As %String(MAXLEN = "")
Property plainText As %String(MAXLEN = "")
Property issueId As %String(MAXLEN = "")
}
Class Messages.SummaryOutput Extends Ens.Response
{
Property summaryText As %String(MAXLEN = "")
}
Class Operations.GenerateSummary Extends Ens.BusinessOperation
{
Property ollama As %SYS.Python
Property json As %SYS.Python
Method GetSummaryFromText(request As Messages.SummaryInput, Output response As Messages.SummaryOutput) As %Status
{
#dim sc As %Status = $$$OK
Try {
Set embedding = ..PyTransform(request.plainText)
Set response = ##class(Messages.SummaryOutput).%New()
Set response.summaryText=embedding
set ^zSummary(request.issueId)=embedding
} Catch ex {
Set sc = ex.AsStatus()
}
Return sc
}
Method OnInit() As %Status
{
#dim sc As %Status = $$$OK
Try {
Do ..PyInit()
} Catch ex {
Set sc = ex.AsStatus()
}
Quit sc
}
Method PyInit() [ Language = python ]
{
import os
import json
import ollama
import sys
os.environ['TRANSFORMERS_CACHE'] = '/caches'
os.environ['HF_HOME'] = '/caches'
os.environ['HOME'] = '/caches'
os.environ['HF_DATASETS_CACHE'] = '/caches'
self.ollama = ollama
self.json = json
}
Method PyTransform(text As %String) As %String [ Language = python ]
{
import os
import json
import ollama
import sys
response = ollama.chat(model='gemma:2b', messages=[
{
'role': 'system',
'content': 'Your goal is to summarize the text given to you in roughly 300 words. It is from a meeting between one or more people. Only output the summary without any additional text. Focus on providing a summary in freeform text with what people said and the action items coming out of it. Give me the following sections: Problem, Solution and Additional Information. Please give only the detail, avoid being polite'
},
{
'role': 'user',
'content': text,
},
])
return response['message']['content']
}
XData MessageMap
{
<MapItems>
<MapItem MessageType="Messages.SummaryInput">
<Method>GetSummaryFromText</Method>
</MapItem>
</MapItems>
}
}
Once we have these classes in place, we can add this Operation to an Interoperability production. Make sure to enable Testing at the Production level, so we can feed in some test conversation data, and check that the model is working. In the example code above, the message allows for the passing of jsonText or plainText. For now, only the plainText is read so we should populate this field in testing. Additionally, we should pass in an IssueId, as this will transparently store the results of Summarisation in IRIS for later review
Let's give this a test:
.png)
And the model gives us in return...
.png)
So, we now have an Operation which can access our local LLM, pass in data and get a response! That was pretty easy, what else can we do? Let's add a second Operation using a different model.
Step 3 - Adding an image classification model
Ollama is able to run a wide range of models seamlessly. Llava (https://llava-vl.github.io/) is a model optimised to analyse visual data such as images. We are able to pass in an array of image data, encoded as Base64, and can then ask the model to analyse the text. In this example, we will just ask it for a basic summary of what it sees, but other use cases could be to extract any text data, compare 2 images for likeness and so on. Before we start, drop to your OS terminal and make sure to run the model once, to download all required files for setup
ollama run llava
As we are working with stream data here, testing is a little more challeging. Typically a stream would be retrieved from somewhere in your codebase, and passed into Python. In this example, I have Base64 encoded my Developer Community avatar as it's small enough to embed in the source file. Let's see what Llava has to say about this image
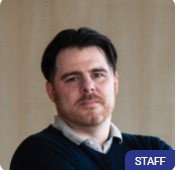
Class Operations.ClassifyImage Extends Ens.BusinessOperation
{
Property ollama As %SYS.Python
Property json As %SYS.Python
Method GetImageSummary(request As Messages.SummaryInput, Output response As Messages.SummaryOutput) As %Status
{
#dim sc As %Status = $$$OK
Try {
set stream = ##class(Issues.Streams).GetStreamByIssueId(request.issueId)
Set embedding = ..PyTransform(stream)
$$$TRACE(embedding)
Set response = ##class(Messages.SummaryOutput).%New()
Set response.summaryText=embedding
} Catch ex {
Set sc = ex.AsStatus()
}
Return sc
}
Method OnInit() As %Status
{
#dim sc As %Status = $$$OK
Try {
Do ..PyInit()
} Catch ex {
Set sc = ex.AsStatus()
}
Quit sc
}
Method PyInit() [ Language = python ]
{
import os
import json
import ollama
import sys
os.environ['TRANSFORMERS_CACHE'] = '/caches'
os.environ['HF_HOME'] = '/caches'
os.environ['HOME'] = '/caches'
os.environ['HF_DATASETS_CACHE'] = '/caches'
self.ollama = ollama
self.json = json
}
Method PyTransform(image As %Stream.GlobalBinary) As %String [ Language = python ]
{
import os
import json
import ollama
import sys
## We would normally pass in the stream from the image paramater, but this is hardcoded here for ease of testing
response = ollama.chat(model='llava', messages=[
{
"role": "user",
"content": "what is in this image?",
"images": ["/9j/4AAQSkZJRgABAQEAYAB... Snipped for brevity"]
}
]
)
return response['message']['content']
}
XData MessageMap
{
<MapItems>
<MapItem MessageType="Messages.SummaryInput">
<Method>GetImageSummary</Method>
</MapItem>
</MapItems>
}
}
Once we have run this using the Test Harness, we get a plaintext summary returned
.png)
This has done a pretty decent job of describing this image (leaving aside 'middle-aged', obviously). It has correctly classified the main aspects of my appearance, and has also extracted the presence of the word "STAFF" within the image.
So, with just 4 classes and a couple of external packages installed, we now have the ability to access 2 different LLM models from within IRIS Interoperability. These Operations are available to use by another other code running on the system, simply by invoking the Operations with the defined messaging types. The calling code does not need any special modification in order to leverage the output of the LLMs, plain text is returned and all of the complex plumbing is abstracted away
Step 4 - What's next?
We now have a template to run any models that can be hosted on Ollama (with another reminder that you may need a hefty GPU to run some larger models). These operations are intentionally very simple, so you can use them as building blocks for your own use cases. What else could you do next? Here's some ideas
Example code available at: https://github.com/iscChris/LLMQuickStart. Note that the Docker image does not build with Ollama (sorry, I'm bad at Docker), but the code will work on a properly configured instance (I'm using WSL)