Over the past couple of months, I have been working on the SMART on FHIR EHR Launch to test the capabilities of IRIS for Health using two open-source apps from CSIRO: SMART-EHR-Launcher and SMART Forms App. This journey has been incredibly interesting, and I’m truly grateful for the opportunity to work on this task and explore more of IRIS for Health’s potential.
After successfully demonstrating the seamless launch of multiple external SMART apps at the HL7 AU FHIR Connectathon, I’m excited to share what I’ve learned with the community. I hope my insights can help others get a faster start on similar projects.
The task involved using SMART-EHR-Launcher as the EHR to launch the SMART Forms App. Simultaneously, IRIS for Health was utilized as the FHIR repository for the EHR, and its OAuth2 Server was used as the Authorization Server.
Before diving into all the excitement, let’s take a closer look at SMART on FHIR and the SMART on FHIR EHR Launch. Let’s ask our good friend ChatGPT.
What is SMART on FHIR?

What is SMART on FHIR EHR Launch?

From SMART App Launch website (https://build.fhir.org/ig/HL7/smart-app-launch/app-launch.html) we can find the SMART App Launch details.
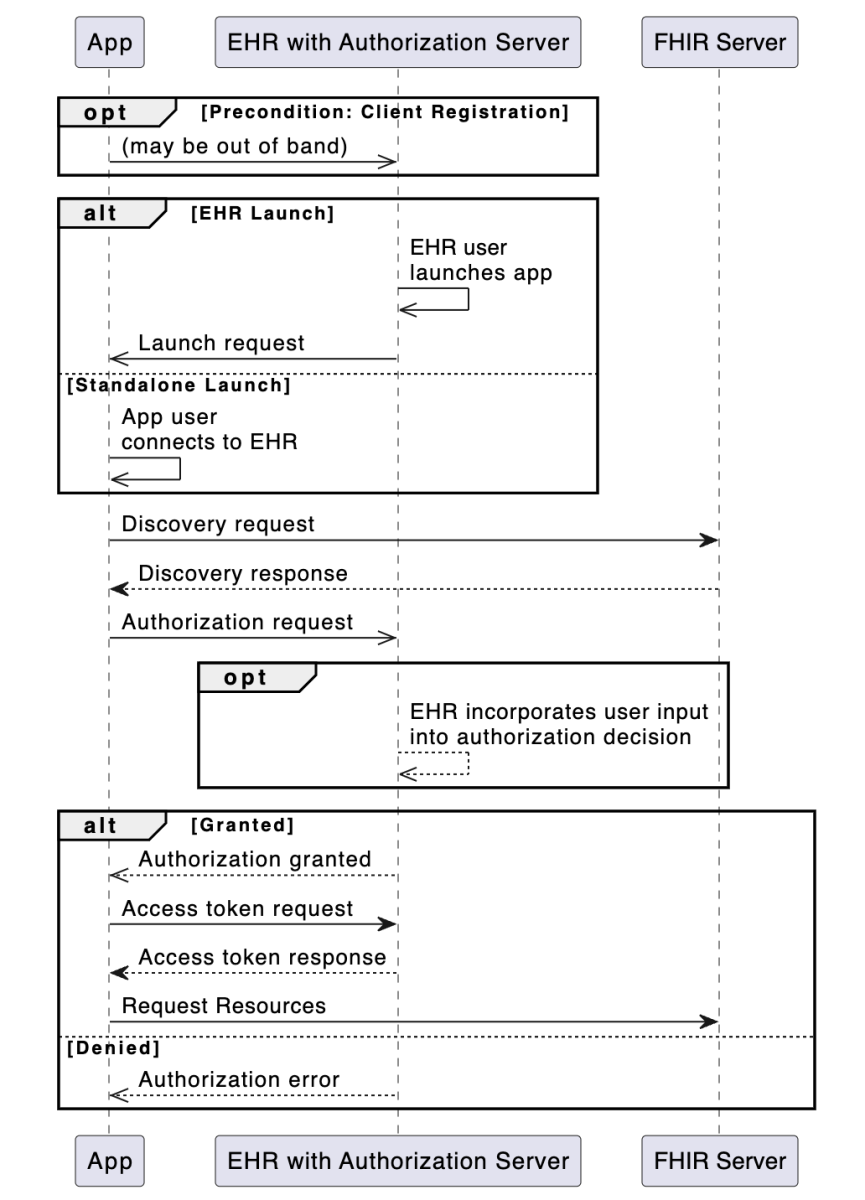
Now, let the game begin!
Applications Deployment and Configurations
SMART-EHR-Launcher: Follow the steps in the GitHub repository to deploy SMART-EHR-Launcher. Ensure that you configure the app's .env
file by setting VITE_FHIR_SERVER_URL
to the IRIS for Health FHIR server endpoint.
Once deployed, open the app and click the arrow next to the practitioner.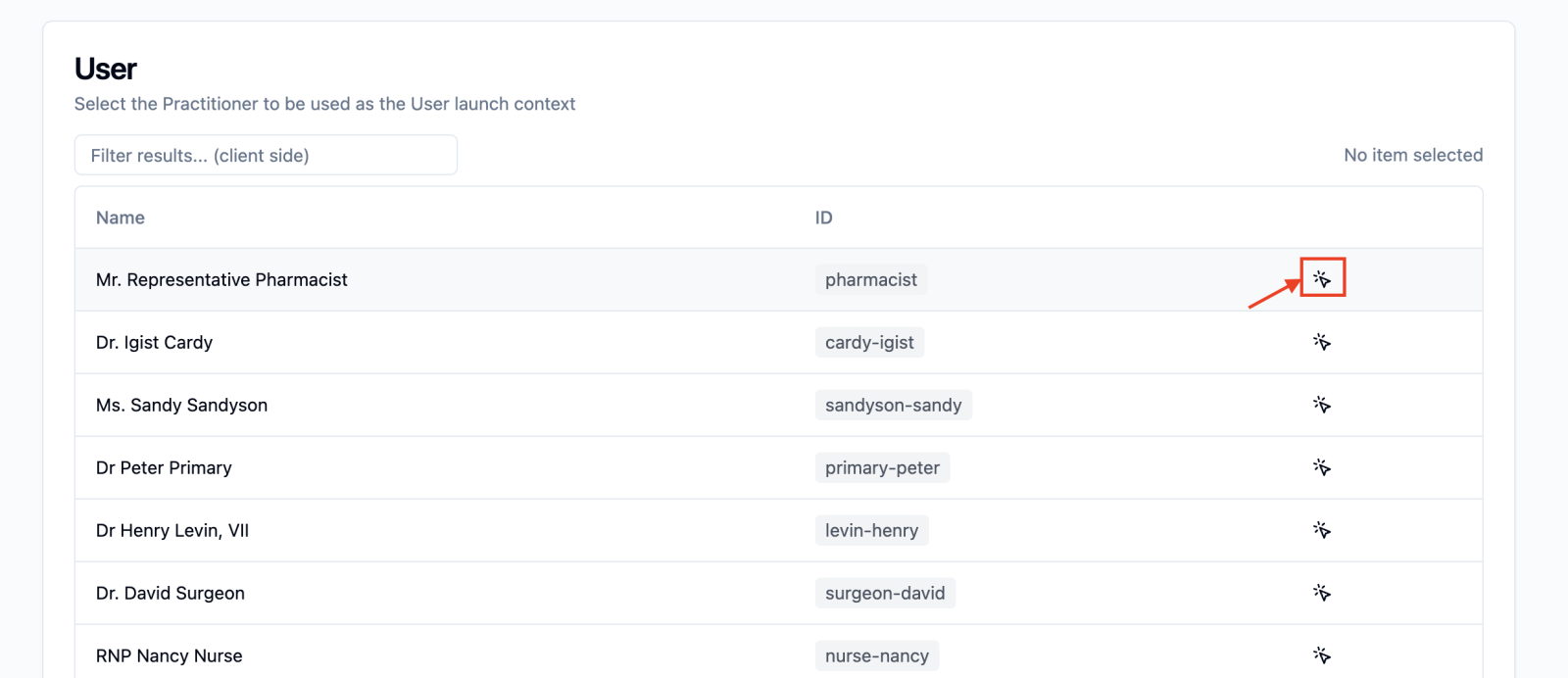
Next, click the arrow next to the patient.
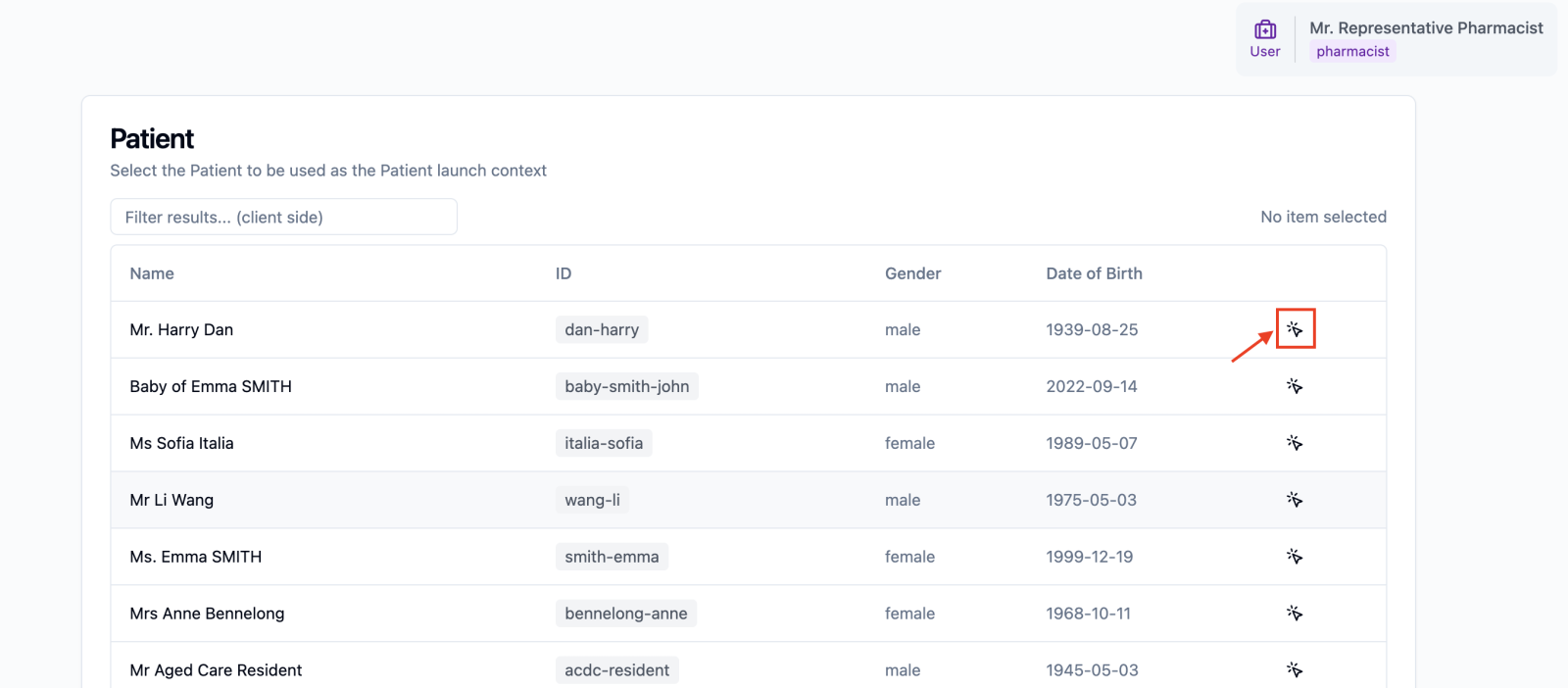
Finally, we can access the launch button.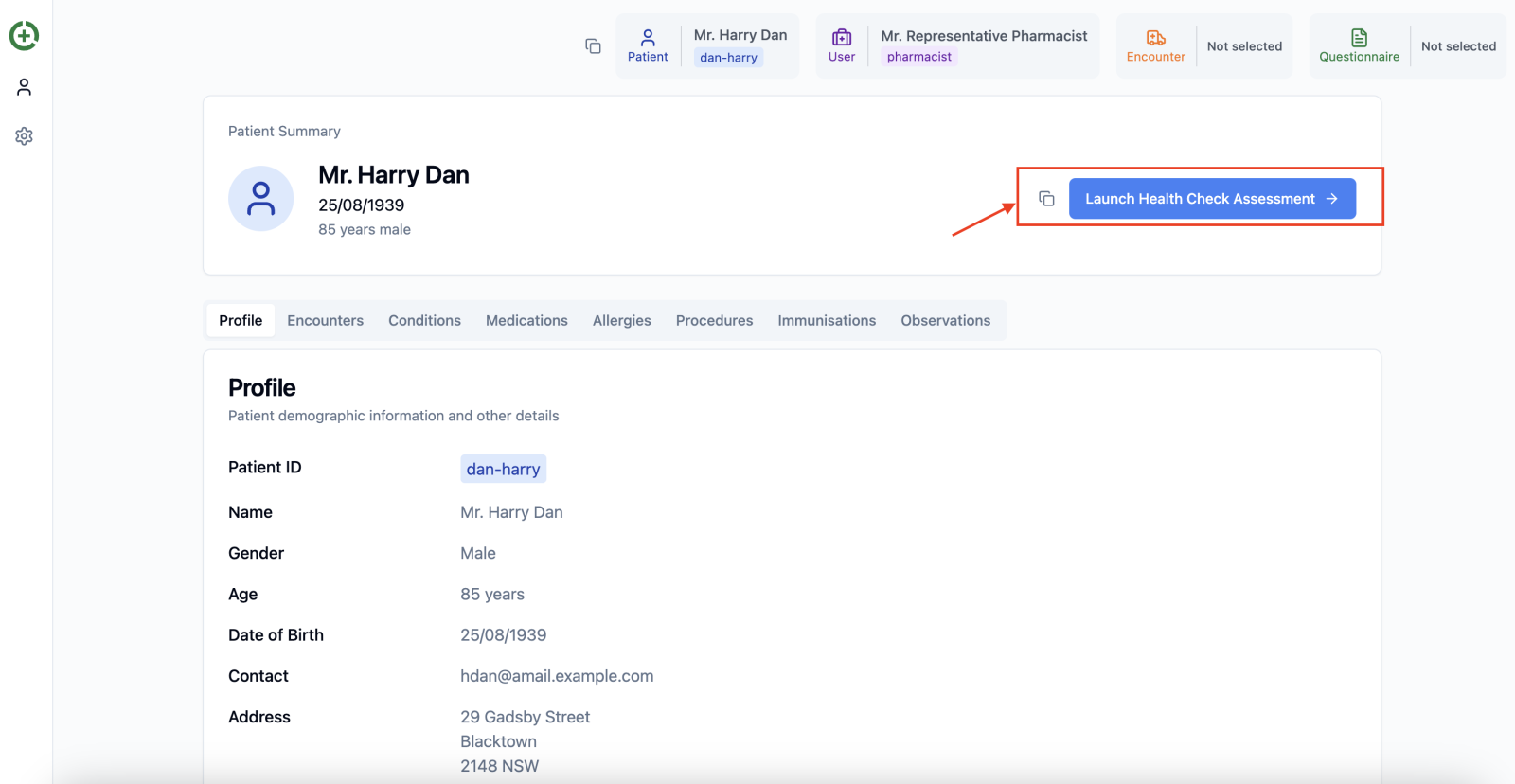
Let’s take a closer look at the launch button URL: https://localhost:5173/launch?iss=https%3A%2F%2Fmyfhirserver%2Fr4&launch=xyz123
To launch the SMART app from the EHR, two parameters are required: iss
and launch
.
- iss dentifies the EHR's FHIR endpoint, which the app can use to obtain additional details about the EHR including its authorization URL.
- Launch is an opaque identifier for this specific launch and any EHR context associated with it. This parameter must be communicated back to the EHR at authorization time by passing along a launch parameter.
SMART Forms: Follow the steps in the GitHub repository to deploy SMART Forms. Ensure that the webserver supports HTTP (SSL/TLS ) to enable the secure communication, such as https://localhost:5173
- Update the scope configuration: Check the
.env
file and ensure it includes user/Practitioner.rs
. If it is missing, add it to the scope.
- Library usage: This app utilizes the fhirclient.js library to manage SMART on FHIR processes. For more information, visit fhirclient.js documentation.
IRIS OAuth2 configurations
SMART on FHIR uses OAuth 2.0 for secure authorization. Let’s explore how to configure OAuth 2.0 on IRIS for Health. There are excellent articles and videos available for a deeper dive into OAuth 2.0 on IRIS. If you're interested, feel free to check out this article and this video.
In this article, I will focus specifically on configuring OAuth 2.0 on IRIS to support the SMART App Launch framework.
In this example, IRIS is the authorization server and the resource server. SMART Forms is the client application, it will be registered in the IRIA OAuth2 client registration.
IRIS OAuth2 Authorization Server configuration
Navigate to IRIS for Health Management Portal -> System Administration -> Security -> OAuth 2.0 -> Server
- Enter a Description for the OAuth server.
- Configure the Issuer Endpoint, which allows the authorization server to reference itself. The generated URL will be used later during client configuration.
- Enable PKCE (Proof Key for Code Exchange) for public clients.
- Select the appropriate Grant Types. For the SMART App Launch, ensure that the Authorization Code grant type is enabled.
- Set up the SSL/TLS configuration to ensure secure communication.
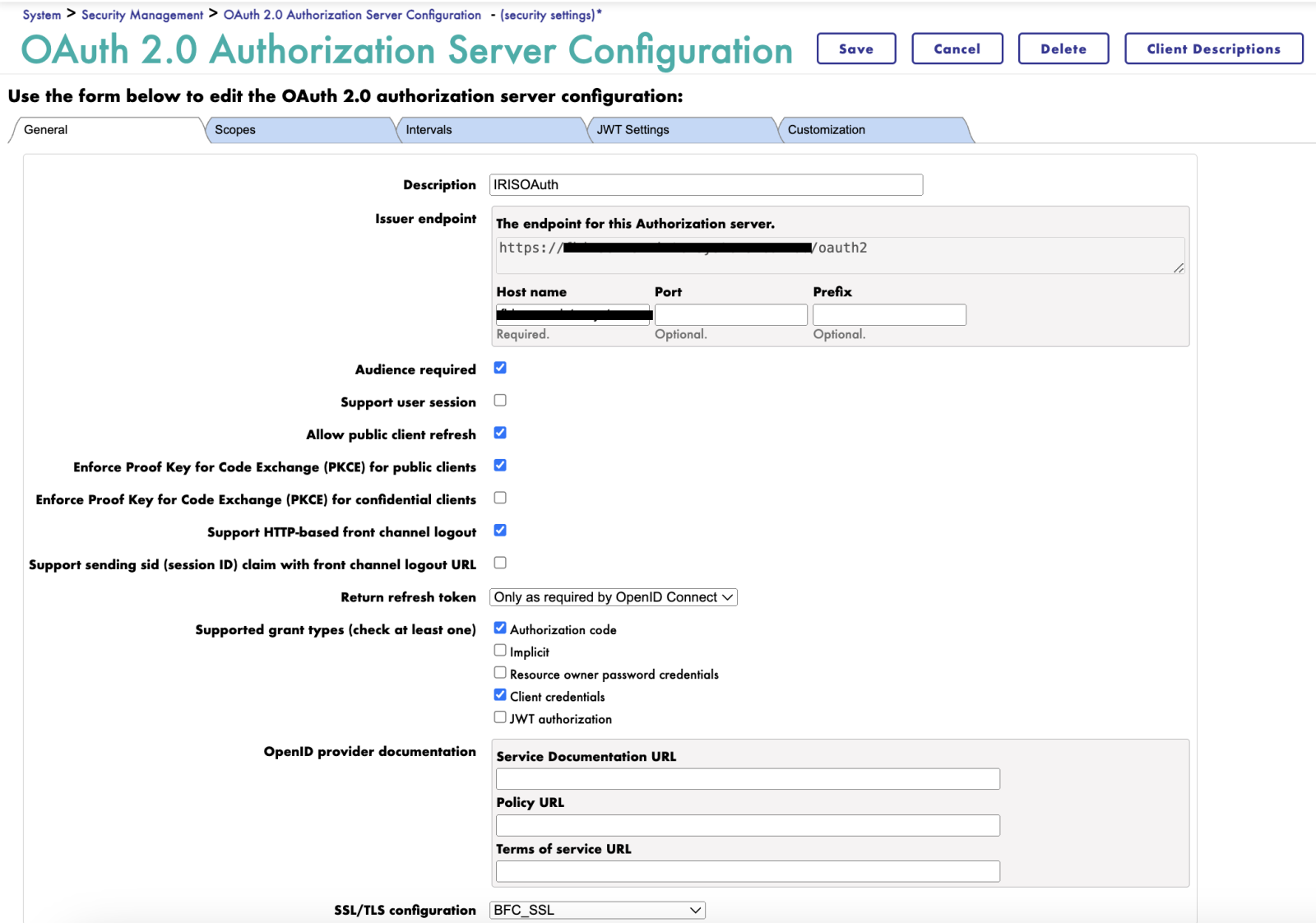
Navigate to the Scopes tab and add the scopes that are relevant to your project.
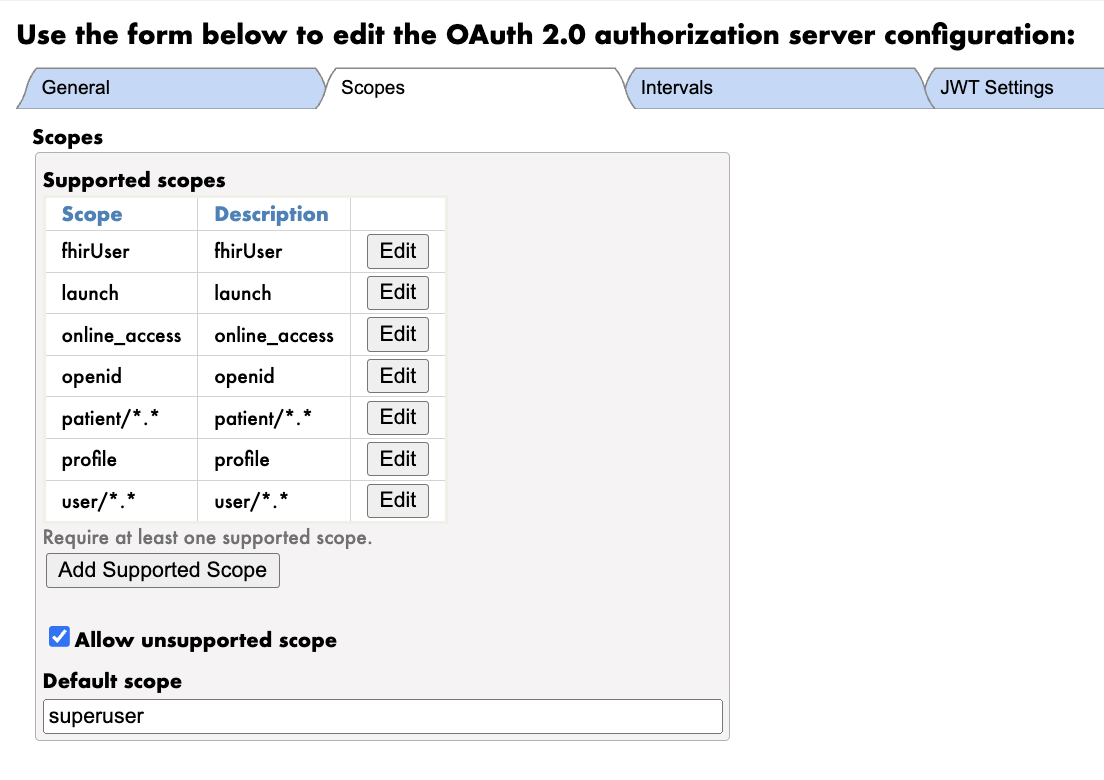
Navigate to the JWT Settings tab. Select a default signing algorithm. If the client-side setting is not specified, the server-side configuration will be used.

Navigate to the Customization tab.
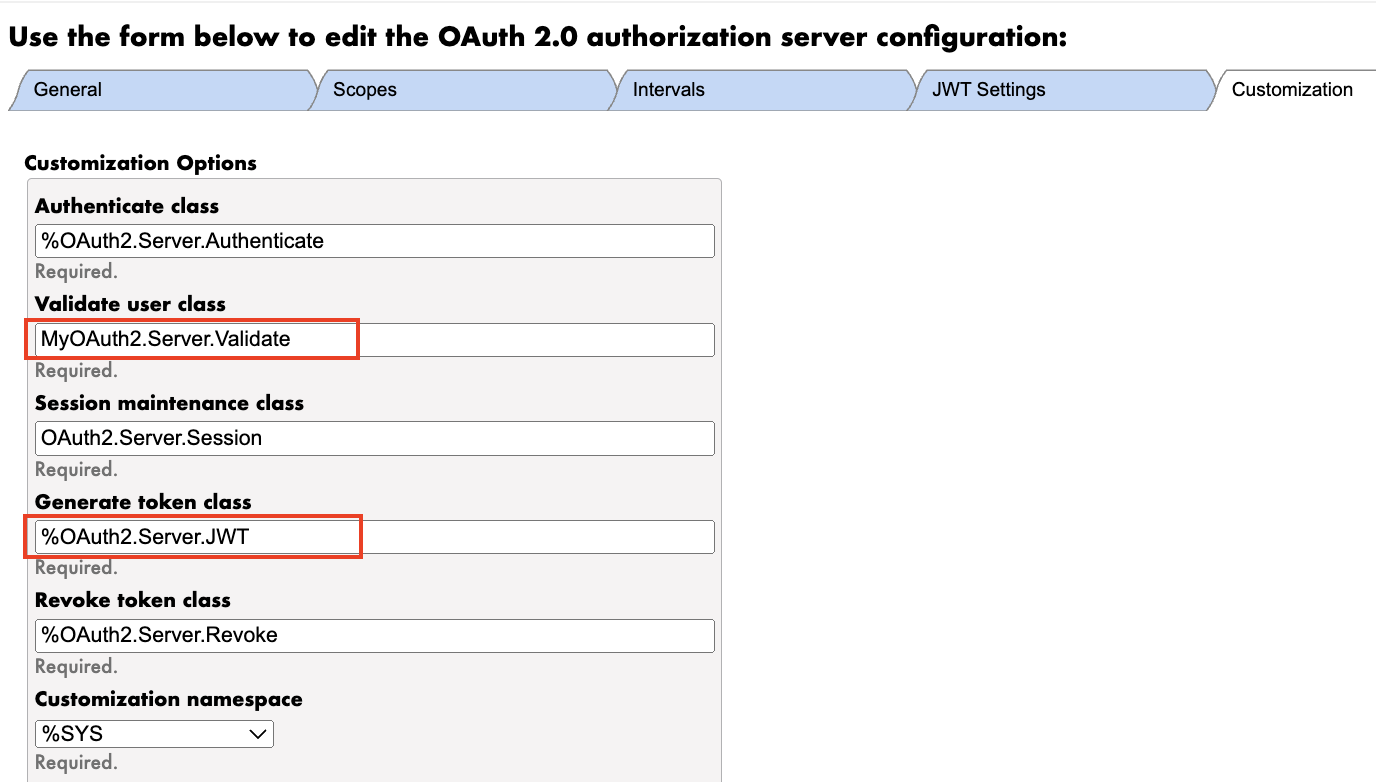
- Authentication Class: This defines the login screen and can be customized to suit the organization’s needs. (Since this is not my primary focus, I’ve left it as is.)
- Validate User Class: This class validates the user’s username and password, as well as the scopes and token information. (Similarly, user authentication and custom scope validation are not my primary focus, so I’ve left them unchanged. For your projects, you should implement the appropriate logic to meet your specific requirements.)
However, to ensure the token contains the correct information for the SMART on FHIR launch, we need to create a custom Validate class (e.g., MyOAuth2.Server.Validate
).
This class should:
- Extend
%OAuth2.Server.Validate
- Override the class method
ValidateUser
- Add additional claims required for the implementation
Code Example:
Set launch = $get(%request.Data("launch",1))
<<Logic to get patient and practitioner ids>>
Do properties.SetClaimValue("profile","Practitioner/"_practitioner,"string")
Do properties.SetClaimValue("fhirUser","Practitioner/"_practitioner,"string")
Do properties.SetClaimValue("patient",patient,"string")
Set claim=##class(%OAuth2.Server.Claim).%New()
If (properties.IDTokenClaims) {
Do properties.IDTokenClaims.SetAt(claim,"profile")
}
Do properties.ResponseProperties.SetAt(patient,"patient")
- Update the Generate Token Class to
%OAuth2.Server.JWT
IRIS OAuth2 Client configuration
Navigate to IRIS for Health Management Portal -> System Administration -> Security -> OAuth 2.0 ->Client
- Click Create Server Description.
- Enter the Issuer Endpoint generated from the OAuth2 Server configuration. (Open the OAuth2 Server configuration and copy the endpoint.)
- Select the appropriate SSL/TLS configuration.
- Click Discover and Save.
- All the endpoints are displayed.
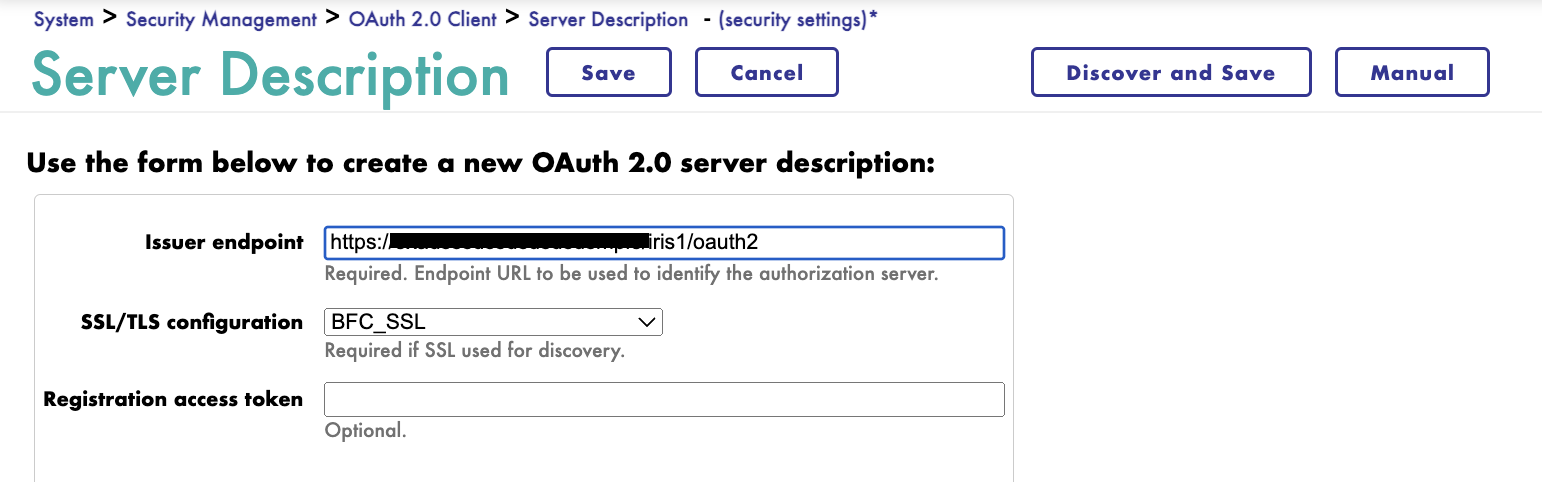
Navigate back to the previous screen and click Client Configuration. Next, we will create a public client.
Create a public client:
- Enter the Application Name, Client Name, and Description.
- Enable the client.
- Select Client Type as "Public".
- Choose the appropriate SSL/TLS Configuration.
- Enter the Host Name.
- Select the Required grant type as "Authorization Code"
- Select “form encoded body” as the Authentication type.
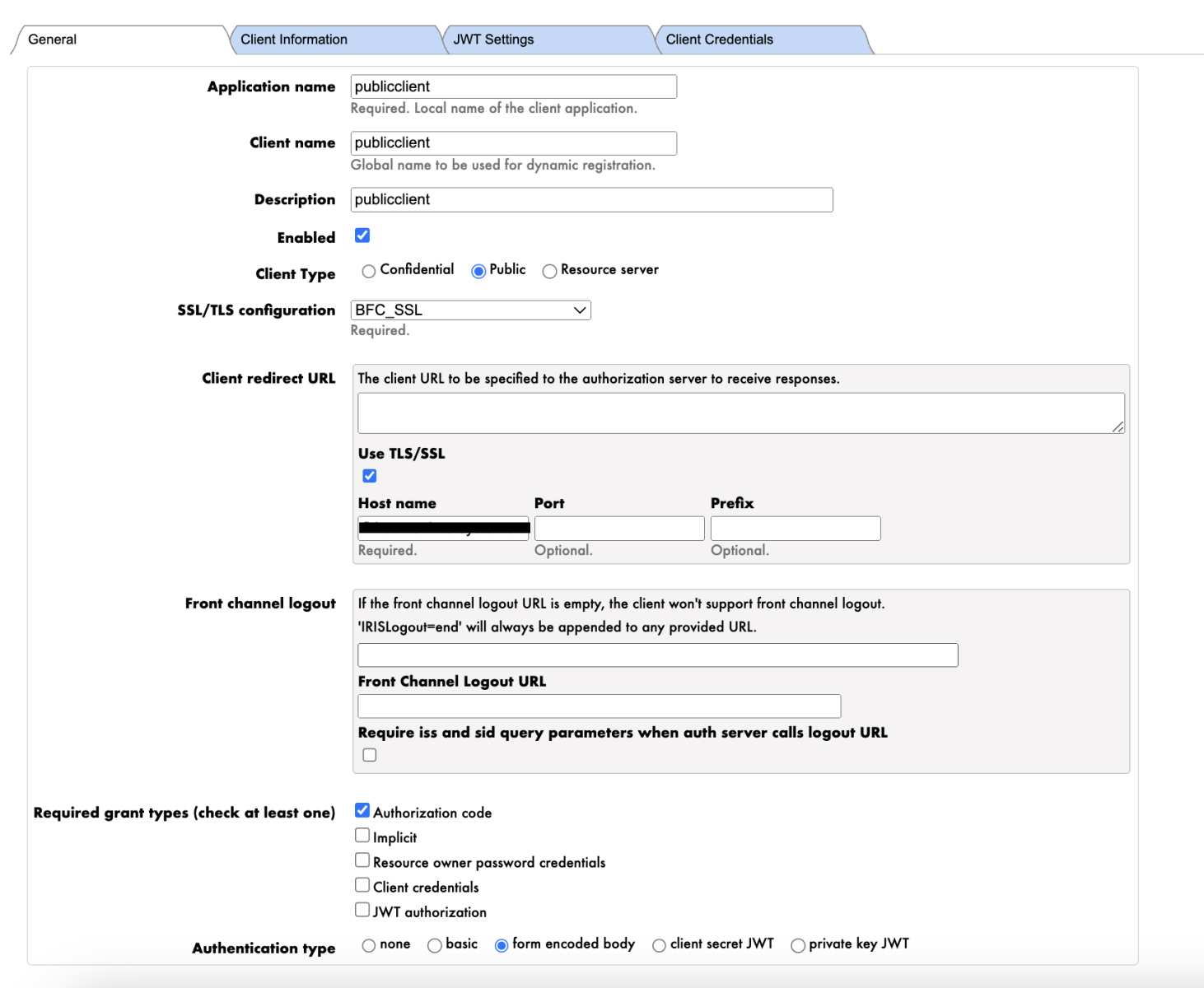
- In JWT Settings, select an Access token algorithm.
- Click Dynamic Registration and Save.
- A Client ID is generated. (Note: There is no secret for a public client.)
- Navigate to IRIS for Health Management Portal -> System Administration -> Security -> OAuth 2.0 -> Server.
- Click Client Description and open the public client.
- Add https://localhost:5173/ to the Redirect URLs.
- Click Save.
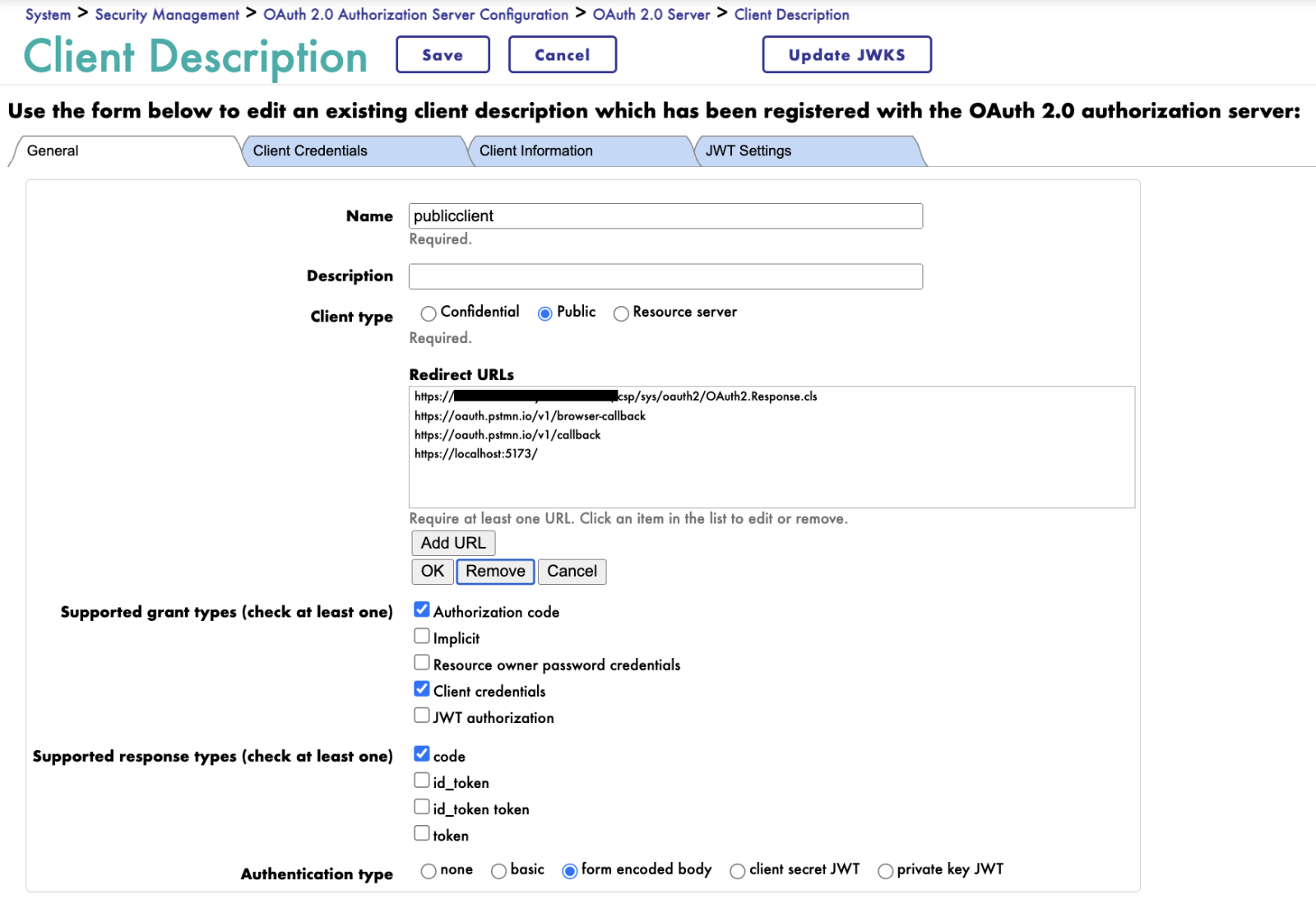
IRIS OAuth2 Resource Server configuration
Navigate to IRIS for Health Management Portal -> System Administration -> Security -> OAuth 2.0 ->Client.
Click Client Configuration as in the previous setup. This time, we will create a resource server (client).
- Enter the Application Name, Client Name, and Description.
- Enable the client.
- Choose the Client Type as Resource Server.
- Select the appropriate SSL/TLS Configuration.
- Set the Authentication Type to basic.
- Click Dynamic Registration and Save.
- A Client ID and Client Secret are generated.
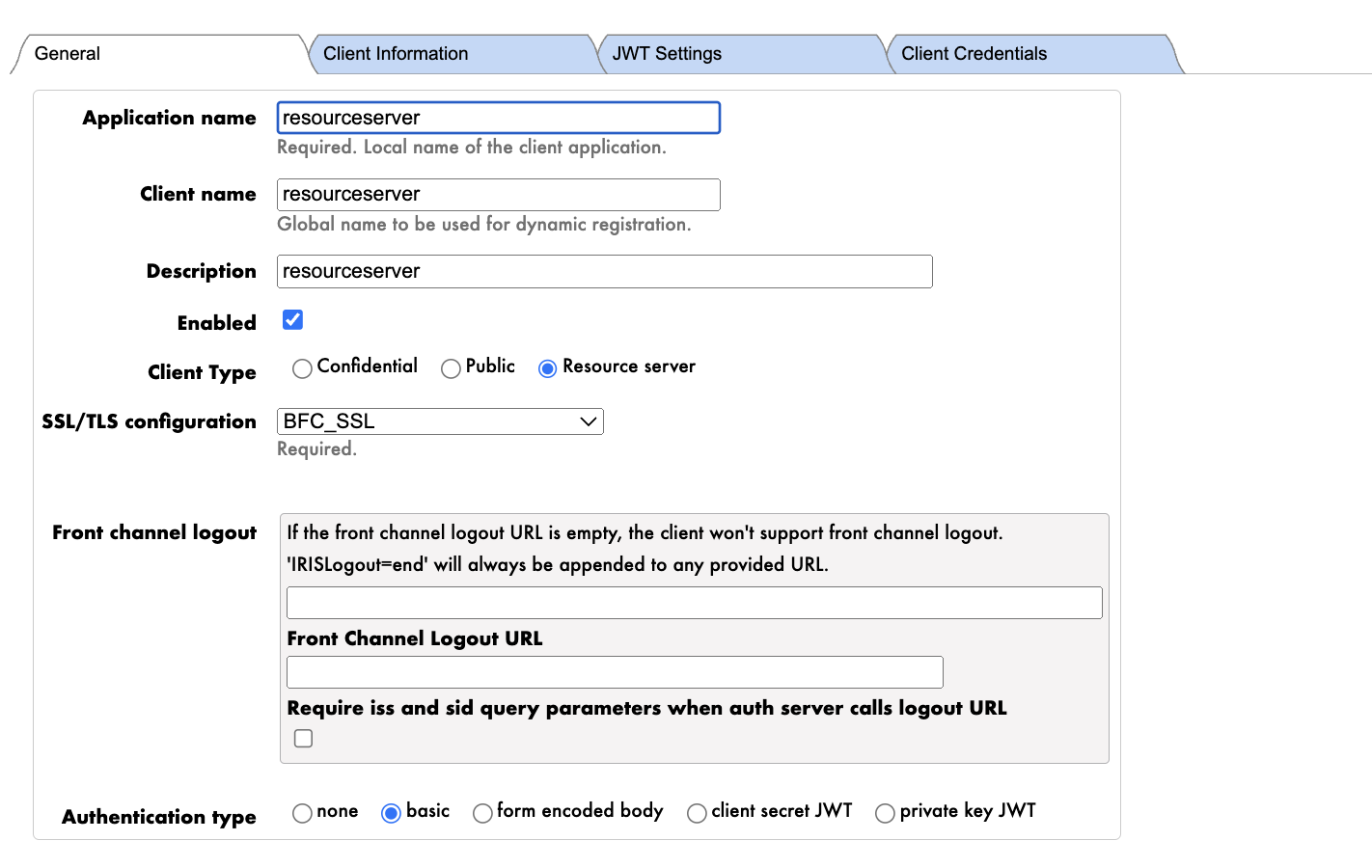
Configure the FHIR Server
Navigate to the IRIS for Health Management Portal: Health → Namespace → FHIR Configuration → Server Configuration.
Enter the OAuth Client Name as shown in the following screenshot, then save the changes.
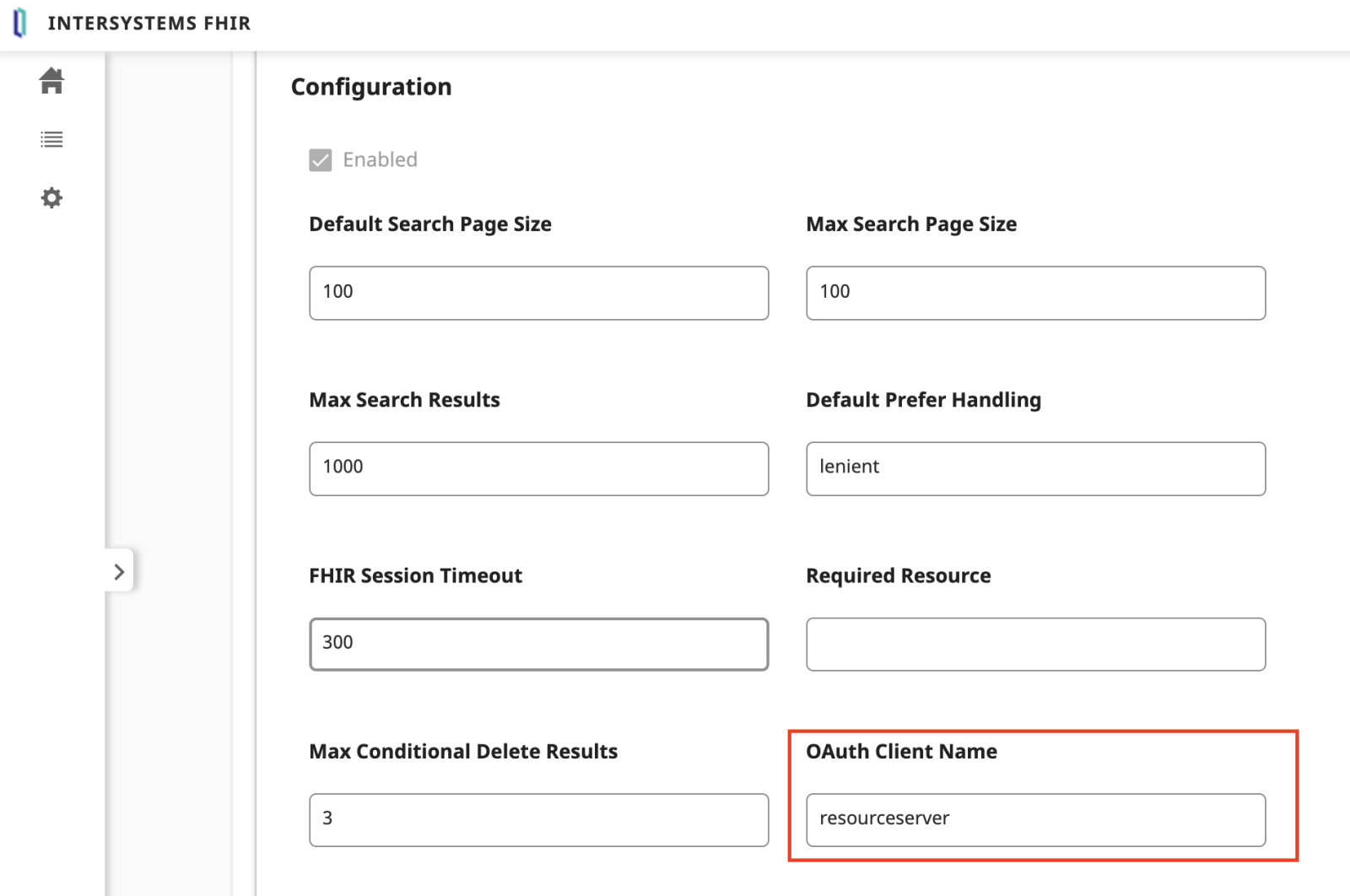
Launch!
With all the configurations complete, it’s time to click the Launch button in the EHR. 
The generic IRIS verification screen opens.
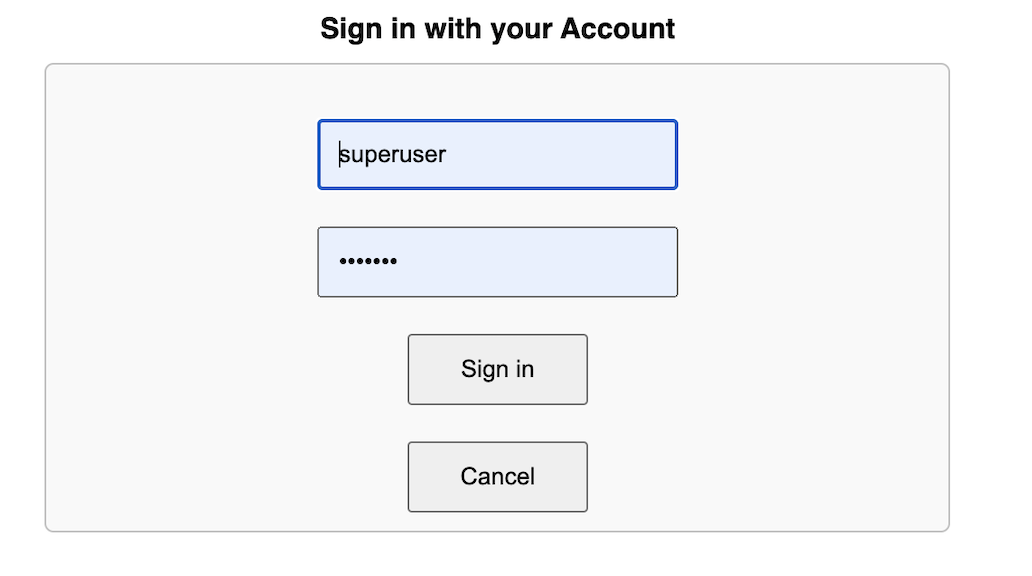
After clicking Sign In, a list of scopes is displayed.
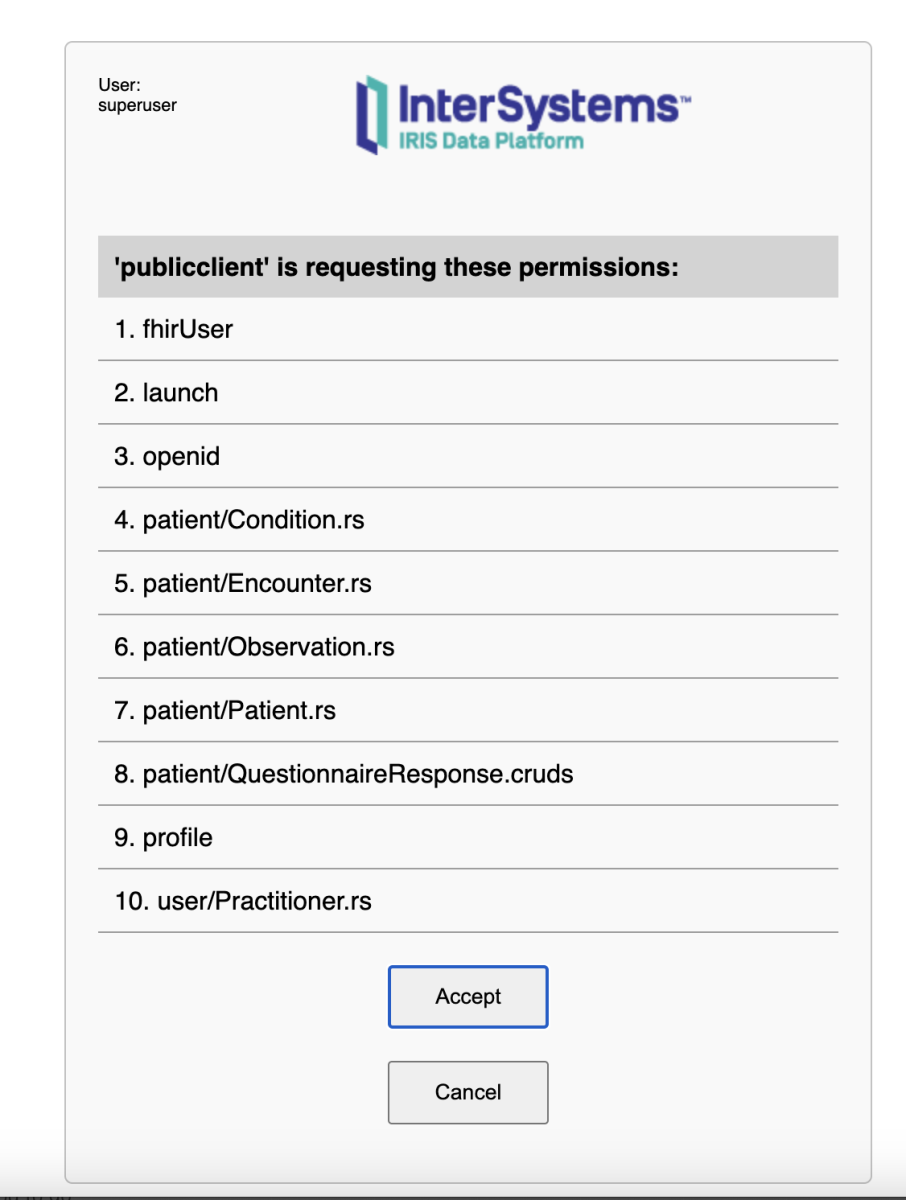
After selecting Accept to grant access, the Questionnaires page appears. The SMART Forms app successfully retrieves and displays the Patient and Practitioner data from the EHR FHIR repository.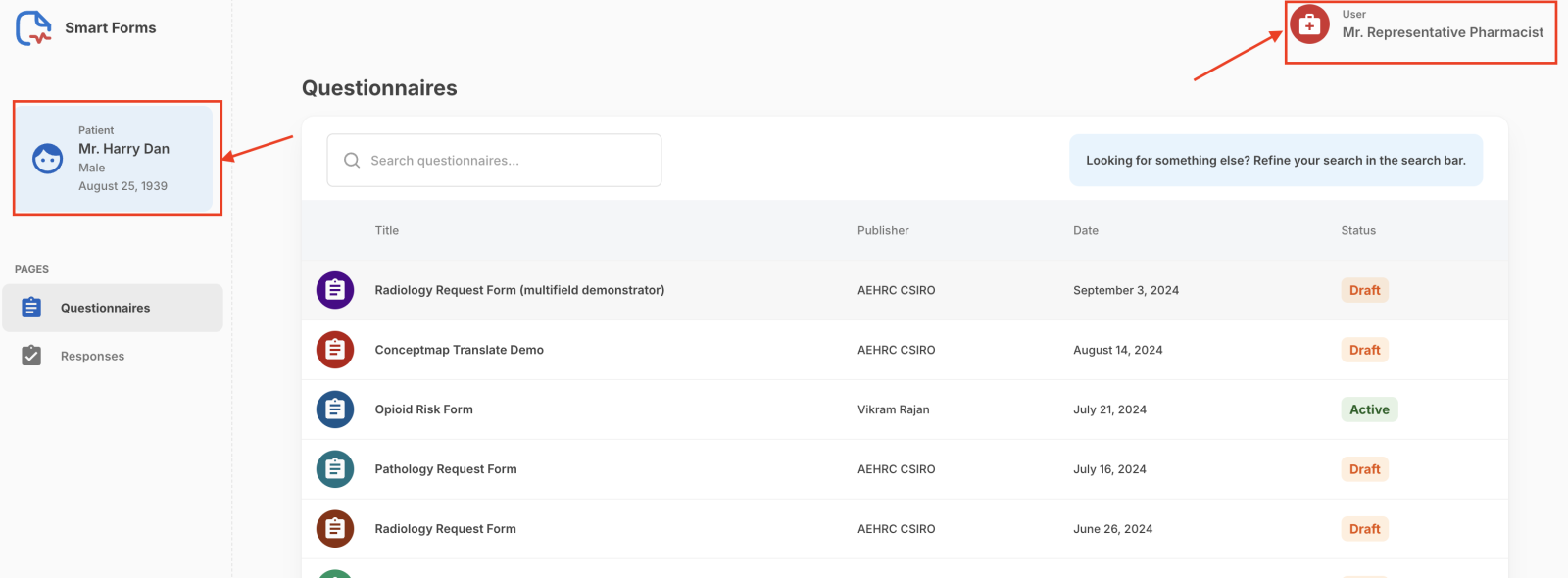
Thank you for following along with this guide on the SMART on FHIR EHR Launch with IRIS for Health. I hope it has provided you with the knowledge and tools to get started on your own projects. Best of luck, and enjoy exploring the possibilities!